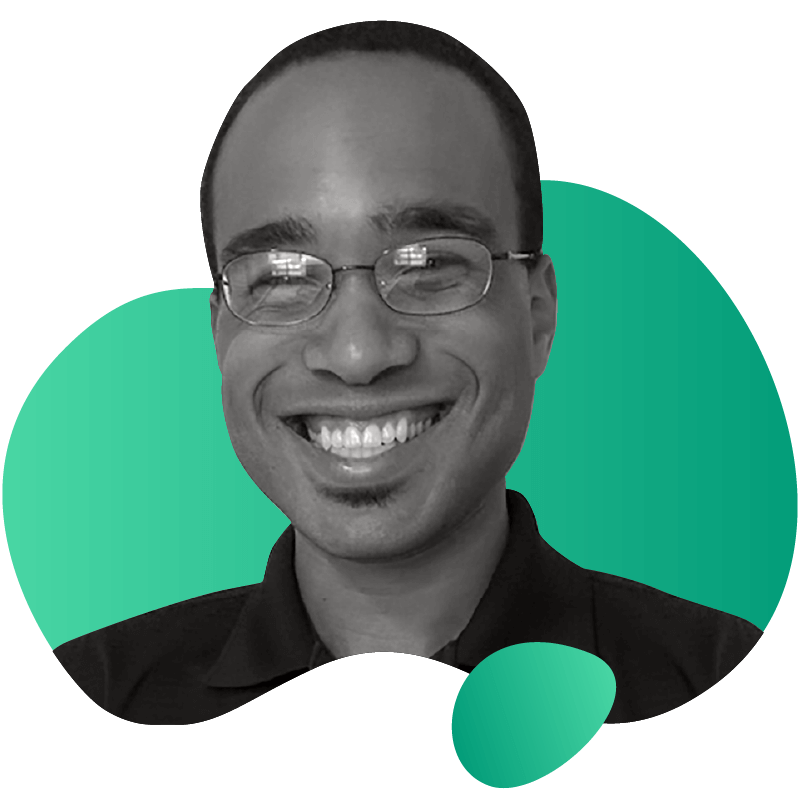
In this article, we take an in-depth look at some of the most popular open source and commercial Chaos Engineering tools available in the community. This isn’t meant to be a direct comparison between other tools and Gremlin, but rather an objective look at each tool’s features, ease of use, system/platform support, and extensibility.
Our team at Gremlin has decades of combined experience implementing Chaos Engineering at companies like Netflix and Amazon. We understand how to apply Chaos Engineering to large-scale systems, and which features engineers are most likely to want in a Chaos Engineering solution. We’ll provide a table at the end so you can see how these tools compare.
Platform: Spinnaker
Release year: 2012
Creator: Netflix
Language: Go
No Chaos Engineering tool list is complete without Chaos Monkey. It was one of the first open source Chaos Engineering tools and arguably kickstarted the adoption of Chaos Engineering outside of large companies. From it, Netflix built out an entire suite of failure injection tools called the Simian Army, although many of these tools have since been retired or rolled into other tools like Swabbie.
Chaos Monkey is deliberately unpredictable. It only has one attack type: terminating virtual machine instances. You set a general time frame for it to run, and at some point during that time it will terminate a random instance. This is meant to help replicate unpredictable production incidents, but it can easily cause more harm than good if you’re not prepared to respond. Fortunately, you can configure Chaos Monkey to check for outages before it runs, but this involves writing custom Go code.
While Chaos Monkey is historically important, its limited number of attacks, lengthy deployment process, Spinnaker requirement, and random approach to failure injection makes it less practical than other tools.
Pros:
Cons:
Platforms: Docker, Kubernetes, bare-metal, cloud platforms
Release year: 2019
Creator: Alibaba
Language: Go
ChaosBlade is built on nearly ten years of failure testing at Alibaba. It supports a wide range of platforms including Kubernetes, cloud platforms, and bare-metal, and provides dozens of attacks including packet loss, process killing, and resource consumption. It also supports application-level fault injection for Java, C++, and Node.js applications, which provides arbitrary code injection, delayed code execution, and modifying memory values.
ChaosBlade is designed to be modular. The core tool is more of an experiment orchestrator, while the actual attacks are performed by separate implementation projects. For example, the chaosblade-exec-os project provides host attacks, the chaosblade-exec-cplus project provides C++ attacks.
Running an experiment is fairly straightforward compared to other tools. Using the ChaosBlade CLI involves:
blade prepare
to run pre-experiment actions (e.g. attaching to a Java Virtual Machine process or C++ application instance).blade create
to start the experiment.blade destroy
to terminate the experiment, which also reverts the impact.For example, here we ran a CPU experiment and queried the results:
1bash-4.4# blade c cpu fullload2{"code":200,"success":true,"result":"06519262501a6b95"}3bash-4.4# blade status 06519262501a6b954{5 "code": 200,6 "success": true,7 "result": {8 "Uid": "06519262501a6b95",9 "Command": "cpu",10 "SubCommand": "fullload",11 "Flag": "--debug false --help false",12 "Status": "Success",13 "Error": "",14 "CreateTime": "2020-11-03T20:34:34.766267Z",15 "UpdateTime": "2020-11-03T20:34:35.8329665Z"16 }17}18bash-4.4# blade d 06519262501a6b9519{"code":200,"success":true,"result":"command: cpu fullload --debug false --help false"}
With the chaosblade-operator project, you can also deploy ChaosBlade to Kubernetes clusters and manage experiments using Custom Resource Definitions (CRDs).
Unfortunately for English speakers, most of ChaosBlade’s documentation is only in Standard Chinese. However, the CLI has English instructions, so you can still run blade --help
to learn how to design and run experiments.
ChaosBlade is a versatile tool supporting a wide range of experiment types and target platforms. However, it lacks some useful features such as centralized reporting, experiment scheduling, target randomization, and health checks. It’s a great tool if you’re new to Chaos Engineering and want to experiment with different attacks.
Pros:
Cons:
Platform: Kubernetes
Release year: 2020
Creator: PingCAP
Language: Go
Chaos Mesh is a Kubernetes-native tool that lets you deploy and manage your experiments as Kubernetes resources. It’s also a Cloud Native Computing Foundation (CNCF) sandbox project.
Chaos Mesh supports 17 unique attacks including resource consumption, network latency, packet loss, bandwidth restriction, disk I/O latency, system time manipulation, and even kernel panics. Since this is a Kubernetes tool, you can fine-tune your blast radius using Kubernetes labels and selectors.
Chaos Mesh is one of the few open source tools to include a fully-featured web user interface (UI) called the Chaos Dashboard. In addition to creating new experiments, you can use the Dashboard to manage running experiments and view a timeline of executions. Chaos Mesh also integrates with Grafana so you can view your executions alongside your cluster’s metrics to see the direct impact.
You can run experiments immediately, or use cron to schedule them. Note that Chaos Mesh doesn’t have a built-in scheduling mechanism, and by default, ad-hoc experiments run indefinitely. The only way to set a duration for an experiment is either by scheduling it, or by manually stopping it.
Lastly, Chaos Mesh provides a GitHub Action that lets you integrate experiments into your CI/CD pipeline. This creates a temporary Kubernetes cluster using Kubernetes in Docker (kind), deploys your application and Chaos Mesh, and runs a command that you specify for validation. Other tools can support CI/CD integration, but this is a convenient shortcut if you already host your code on GitHub.
Chaos Mesh offers one approach to Chaos Engineering on Kubernetes through its Dashboard and configuration structure. It provides a deployment path and encourages automated chaos experiments through CI/CD. However, its biggest limitations are its lack of node-level experiments, lack of native scheduling, and lack of time limits on ad-hoc experiments.
Pros:
Cons:
Platforms: Kubernetes
Release year: 2018
Creator: MayaData
Language: TypeScript
Like Chaos Mesh, Litmus is a Kubernetes-native tool that is also a CNCF sandbox project. It was originally created for testing OpenEBS, an open source storage solution for Kubernetes. It provides a large number of experiments for testing containers, Pods, and nodes, as well as specific platforms and tools. For example, you can terminate Amazon EC2 instances, simulate a disk failure in a Kafka cluster, or kill an OpenEBS Pod. Each experiment is fully documented with step-by-step instructions, and Litmus also provides a centralized public repository of experiments called ChaosHub that anyone can contribute experiments to.
Litmus includes a health checking feature called Litmus Probes, which lets you monitor the health of your application before, during, and after an experiment. Probes can run shell commands, send HTTP requests, or run Kubernetes commands to check the state of your environment before running an experiment. This is useful for automating error detection and halting an experiment if your systems are unsteady.
However, getting started with Litmus is much harder than with most other tools. By default, Litmus requires you to create service accounts and annotations for each application and namespace that you want to experiment on (you can reduce some of this managerial overhead by running Litmus in administrator mode, which consolidates resources into a single namespace). While this adds security and makes it harder to cause an accidental failure, it also complicates experiments. Running a Litmus experiment involves:
Deploying the Chaos Chart containing your experiment. This creates a chaosexperiment
resource.
Creating a chaosServiceAccount
to allow Litmus to run in your application’s namespace.
Annotating your application to allow Litmus to run experiments.
Creating a ChaosEngine
resource to connect your application to the experiment. This is also where you set the experiment’s parameters.
ChaosEngine
resource.Waiting for the experiment to generate a chaosresult
, which tells you the status of the experiment.
Cleaning up all of these resources when you’re done testing.
Alternatively, you can manage experiments using Litmus Portal, a web-based UI. Litmus Portal uses Workflows, which leverage Argo to run multiple attacks in the same experiment. In addition to creating, executing, and scheduling Workflows, Litmus Portal lets you assign a weight to each experiment that indicates the importance of a successful outcome. When the experiment finishes, this weight is factored into an overall resilience score.
While Litmus is a comprehensive tool with many useful attacks and monitoring features, it comes with a steep learning curve. Simply running an experiment is a multi-step process that involves setting permissions and annotating deployments. Workflows help with this, especially when used through the Litmus Portal, but they still add an extra layer of complexity. This isn’t helped by the fact that some features—like the Litmus Portal itself—don’t appear in the documentation, and are only available through the project’s GitHub repository.
Pros:
Cons:
Platforms: Docker, Kubernetes, bare-metal, cloud platforms
Release year: 2018
Creator: ChaosIQ
Language: Python
Chaos Toolkit will be familiar to anyone who’s used an infrastructure automation tool like Ansible. Instead of making you select from predefined experiments, Chaos Toolkit lets you define your own.
Each experiment consists of Actions and Probes. Actions execute commands on the target system, and Probes compare executed commands against an expected value. For example, we might create an Action that calls stress-ng to consume CPU on a web server, then use a Probe to check whether the website responds within a certain amount of time. Chaos Toolkit also provides drivers for interacting with different platforms and services. For example, you can use the AWS driver to experiment with AWS services, or manage other Chaos Engineering tools like Toxiproxy and Istio. Chaos Toolkit can also autodiscover services in your environment and recommend tailored experiments.
Experiments are defined in JSON files and split into three stages:
Each stage can contain multiple Actions and Probes. For example, if we wanted to test our ability to restart a failed Kubernetes Pod, we could create the following experiment:
While Chaos Toolkit supports a number of different platforms, it does run entirely through the CLI. This makes it difficult to run experiments across multiple systems unless you’re using a cloud platform like AWS or an orchestration platform like Kubernetes. Chaos Toolkit also lacks a native scheduling feature, GUI, or REST API.
Few tools are as flexible in how they let you design chaos experiments. Chaos Toolkit gives you full control over how your experiments operate, right down to the commands executed on the target system. But because of this DIY approach, Chaos Toolkit is more of a framework that you need to build on than a ready-to-go Chaos Engineering solution.
Pros:
Cons:
Platforms: Kubernetes
Release year: 2017
Creator: Bloomberg
Language: Python
PowerfulSeal is a CLI tool for running experiments on Kubernetes clusters. Perhaps its most unique feature is that it provides three different run modes:
Experiments (called policies) are defined in YAML files and set the parameters of the experiment, the steps performed, and HTTP probes to check the health of your services. By default, PowerfulSeal continuously runs a policy and only stops on error, unless you specify the number of executions. It also tracks its own internal metrics that can be exported to Prometheus or Datadog, including the number of Pods killed, nodes stopped, and failed executions.
In interactive mode, PowerfulSeal essentially becomes a wrapper around kubectl. You can query your cluster’s resources, terminate Pods, or remove nodes. It’s not as feature-rich as autonomous mode, and is best used for planning experiments or for running small, one-off experiments. Lastly, label mode lets you set per-Pod parameters, such as when the Pod is allowed to be terminated and the probability of it being terminated. This is useful for preventing important Pods from being terminated accidentally.
PowerfulSeal doesn’t offer much that other tools aren’t already capable of. The syntax is easy to understand and the different modes offer some greater control over experiments, but it has a limited number of attack types and lacks other differentiating features.
Pros:
Cons:
Platforms: Any
Release year: 2014
Creator: Shopify
Language: Go
Toxiproxy is a network failure injection tool that lets you create conditions such as latency, connection loss, bandwidth throttling, and packet manipulation. As the name implies, it acts as a proxy that sits between two services and can inject failure directly into traffic.
Toxiproxy has two components: A proxy server written in Go, and a client that communicates with the proxy. When configuring the Toxiproxy server, you define the routes between your applications, then create chaos experiments (called toxics) to alter the behavior of traffic along those routes. You can manage your experiments using a command-line client or REST API.
The main challenge with Toxiproxy is in its design. Because it’s a proxy service, you must reconfigure your applications to route network traffic through it. Not only does this add complexity to your deployments, but it also creates a single point of failure if you have a single server handling multiple applications. For this reason, even the maintainers recommend against using it in production.
Toxiproxy also lacks many controls, such as scheduling experiments, halting experiments, and monitoring. A toxic runs continuously until you delete it, and there’s a risk of intermittent connection errors caused by port conflicts. It’s fine for testing timeouts and retries in development, but not in production.
Pros:
Cons:
Platform: Kubernetes
Release year: 2017
Creators: Google, IBM, and Lyft
Language: Go
Istio is best known as a Kubernetes service mesh, but not many know that it natively supports fault injection. As part of its traffic management feature, Istio can inject latency or HTTP errors into network traffic between any virtual service. Experiments are defined as Kubernetes manifests, making it easy to run and halt experiments. You can choose your targets using existing Istio features, such as virtual services and routing rules, and adjust the magnitude of your attacks to only impact a percentage of traffic. You can also use health checks and Envoy statistics to monitor the impact on your systems.
This is about the extent of Istio’s Chaos Engineering functionality. Experiments can’t be scheduled, executed on hosts, customized extensively, or used outside of Istio. It's more or less taking advantage of Istio's place in the network to perform these experiments without adding any additional Chaos Engineering tools or functionality.
If you already use Istio, this is an easy way to run chaos experiments on your cluster without having to deploy or learn another tool. Otherwise, it’s not worth deploying Istio just for this feature.
Pros:
Cons:
Platforms: Kubernetes
Release year: 2019
Creator: David Zuber (storax)
Language: C++
As if Chaos Engineering wasn’t already fun, now you can play Doom while doing it! Kubedoom is a fork of the classic game, but with a twist: each enemy represents a different Pod in your cluster, and killing an enemy will kill its corresponding Pod. For even more chaos (and with a big enough cluster), you can make each enemy a unique namespace.
Yes! But only if you know you can recover safely. There's no `god mode` cheat code for your cluster.
Pros:
Cons:
Works with: Amazon Relational Database Service (RDS), Elastic Compute Cloud (EC2), Elastic Container Service (ECS), and Elastic Kubernetes Service (EKS)
Release year: 2021
Creator: Amazon Web Services
Announced at AWS re:Invent in late-2020, AWS Fault Injection Simulator (FIS) is a managed service for introducing failure into some of AWS’s most popular services.
FIS supports 7 native attack types including rebooting EC2 instances, draining an ECS
cluster, or rebooting an RDS instance. FIS can also call SSM to run custom commands,
such as using the stress-ng
command to consume resources, or the tc
command to
manage network traffic. Unlike other tools, FIS can inject failure into AWS services
through the AWS control plane. This enables scenarios such as failing over a managed
database cluster or throttling API requests
Each experiment has six components:
Actions can be performed sequentially or in parallel and can target any number of resources. If you’re using SSM, you’ll also need to write an SSM document with the commands you want to run on each target. Stop conditions are defined using CloudWatch alarms, letting you automatically stop attacks.
Since FIS only supports a limited number of AWS services and has a limited number of attacks, whether you use FIS will depend on what services you use in your environment. The process of running an attack in FIS can be difficult. You have to create IAM roles to allow you to run FIS actions, target specific AWS resources by ID, and if using SSM, construct an SSM document. And while the cost of attacking is only $0.10 per minute per action, running long-lived experiments or experiments with multiple actions can quickly add up, plus there are additional costs of using SSM.
Pros:
Cons:
Ultimately, the goal of any Chaos Engineering tool is to help you achieve greater reliability. The question is: which tool will help you achieve that goal faster and more easily? This question of course depends on your tech stack, the experience and expertise of your engineering team, and how much time you can dedicate to testing and evaluating each tool. We put together a comparison matrix to show you how these tools stack up to each other:
Tool | Works with | Total attack types | Application attacks | Host attacks | Container/Pod attacks | GUI | CLI | REST API | Metrics/reporting | Attack sharing | Attack halting | Attack scheduling | Target randomization | Custom attacks | Health checks |
---|---|---|---|---|---|---|---|---|---|---|---|---|---|---|---|
Gremlin | Containers, Kubernetes, bare metal, cloud | 12 | ✔️ | ✔️ | ️✔️ | ✔️ | ✔️ | ️✔️ | ️✔️ | ✔️ | ✔️ | ✔️ | ✔️ | ✔️ | |
Chaos Monkey | Amazon Web Services (requires Spinnaker) | 1 | ✔️ | ️ | ✔️ | ️ | ️ | ✔️ | ✔️ | ✔️ | |||||
ChaosBlade | Containers, Kubernetes, bare metal, cloud | 40 | ✔️ | ✔️ | ✔️ | ✔️ | ✔️ | ✔️ | |||||||
Chaos Mesh | Kubernetes | 17 | ✔️ | ✔️ | ✔️ | ✔️ | ️ | ✔️ | ✔️ | ✔️ | ✔️ | ||||
Litmus | Kubernetes | 39 | ️ | ✔️ | ✔️ | ✔️ | ✔️ | ️ | ✔️ | ✔️ | ✔️ | ✔️ | ✔️ | ✔️ | |
Chaos Toolkit | Containers, Kubernetes, bare metal, cloud | Based on driver | ️ | ✔️ | ✔️ | ️ | ✔️ | ️ | ✔️ | ️ | ️ | ✔️ | ✔️ | ✔️ | |
PowerfulSeal | Kubernetes | 5+️ | ✔️ | ✔️ | ️ | ✔️ | ️ | ✔️ | ️ | ️ | ✔️ | ||||
ToxiProxy | Network | 6 | ✔️️ | ✔️ | ️ | ✔️ | |||||||||
Istio | Kubernetes | 2 | ✔️ | ✔️ | ✔️ | ✔️ | |||||||||
KubeDoom | Kubernetes | 1 | ✔️ | ✔️ | ✔️ | ||||||||||
AWS FIS | Amazon Web Services (RDS, EC2, ECS, EKS) | 7+ | ✔️ | ✔️ | ✔️ | ✔️ | ✔️ | ✔️ | ✔️ | ✔️ |
Gremlin empowers you to proactively root out failure before it causes downtime. See how you can harness chaos to build resilient systems by requesting a demo of Gremlin.
Get started